Modules
Text analytics and natural language processing
Extract insights, triage data, and conduct multilingual search. Our Text Analytics platform provides accurate and comprehensive linguistic analysis of unstructured text to enable more fully informed decision making.
Foundational NLP
High-quality multilingual text analytics and natural language processing clean and prepare unstructured text for searching and advanced analysis.
Key features include:
-
Accurate language identification through use of statistical profiles of each language, and algorithms that detect word and script patterns
-
Tokenization that correctly identifies the boundaries between words using precise linguistic processing and understanding of the text's structure
-
Morphological analysis for breaking words down into their constituent parts to understand their grammatical structure, forms, and inflections
-
Lemmatization to reduce the complexity of textual data by revealing semantically related words from the word base (lemma) using sentence context
-
Part-of-speech tagging that indicates a word’s syntactic role and grammatical category (noun, verb, adjective, etc.)


Entity and Event Extraction
Highly accurate and customizable entity extraction, linking, and disambiguation capabilities detect specific events and relationships within unstructured text.
Key features include:
-
Statistical or deep neural network models (based on computational linguistics and human-annotated training documents), patterns, and exact matching to identify entities in documents
-
Confidence and salience scores to indicate the precision of the match and how important the entity is to the overall document
-
Disambiguation of the identity of similarly named entities mentioned in a document using the Wikidata knowledgebase or internal repositories
-
Coreference resolution to chain together all mentions to an entity within a document
-
Event extraction from key phrases and roles (mentions of entities), based on customer-trained event models
Cross-lingual Search by Meaning
Semantic Similarity enables users to search by key phrase to capture relevant results worded differently or in another language. The outcome is a manageable set of results that focuses on the most relevant information.
Key features include:
-
Uses word embeddings to transform words into vectors: numerical representations that approximate the conceptual distance of one word’s meaning from another
-
Compresses large, complex vectors using meaningful groupings to reduce computing requirements while still making meaningful semantic comparisons
-
Calculates the cosine similarity between word vectors to measure semantic similarity
-
Aligns terms and concepts across each language’s vector space so words can be semantically compared in multiple languages


Understand Sentiment and Concepts
Sentiment Analyzer gleans positive or negative sentiment for uses such as opinion mining, market research, brand monitoring, and executive protection. Topic Extractor helps streamline data analysis by detecting the themes that run throughout the text.
Key features include:
-
Sentiment analysis of text as positive, negative, or neutral, with a confidence score. If the input contains entities, it groups all the relevant sentiment about that entity into one mention.
-
Extraction of the most salient themes of a piece of text, including a list of concepts and key phrases. Concepts may or may not actually appear in the text, while key phrases do and are representative of the overall content.
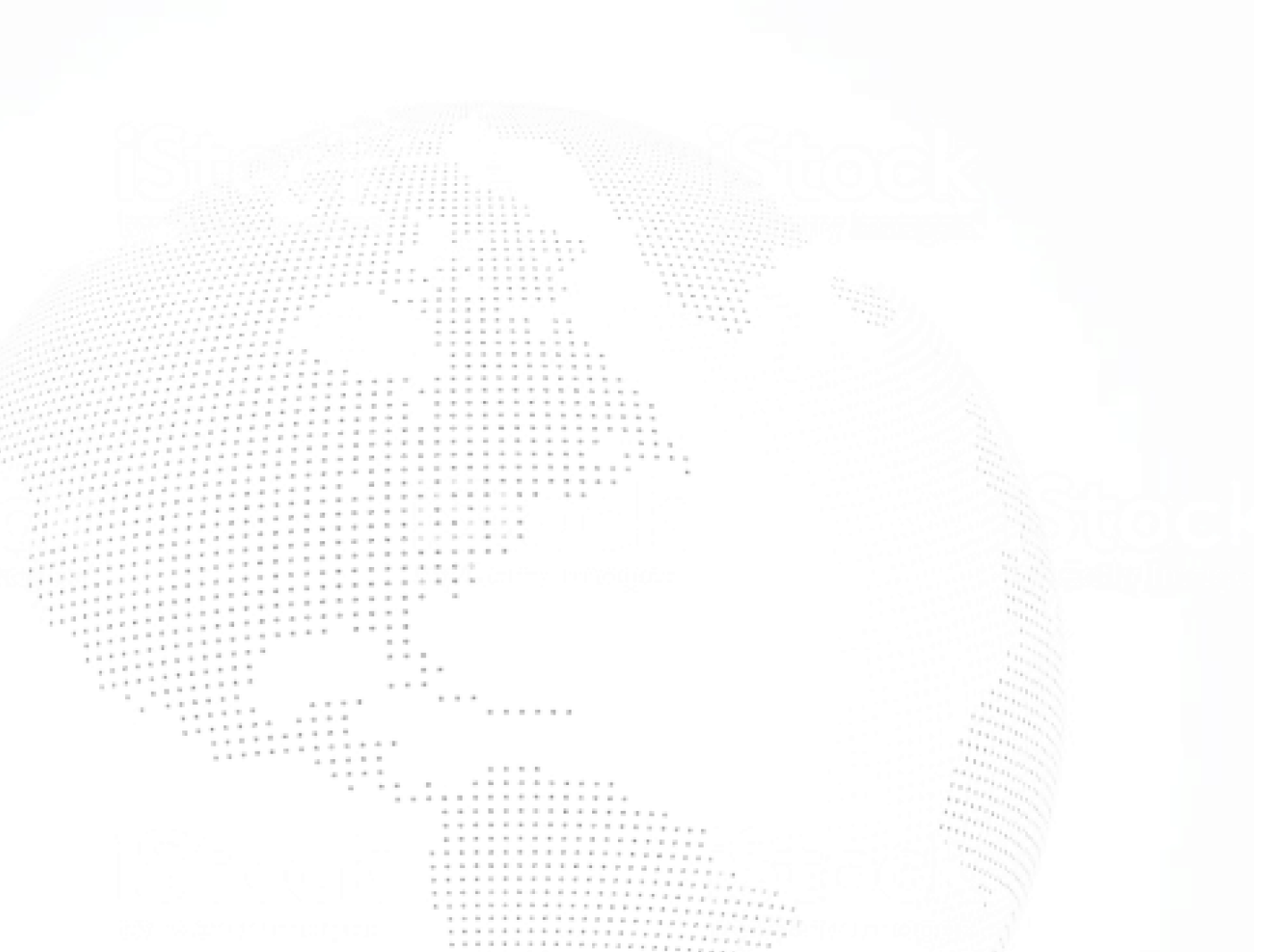
How will you use Babel Street Text Analytics?
Discuss your text analytics and natural language processing requirements with one of our experts.